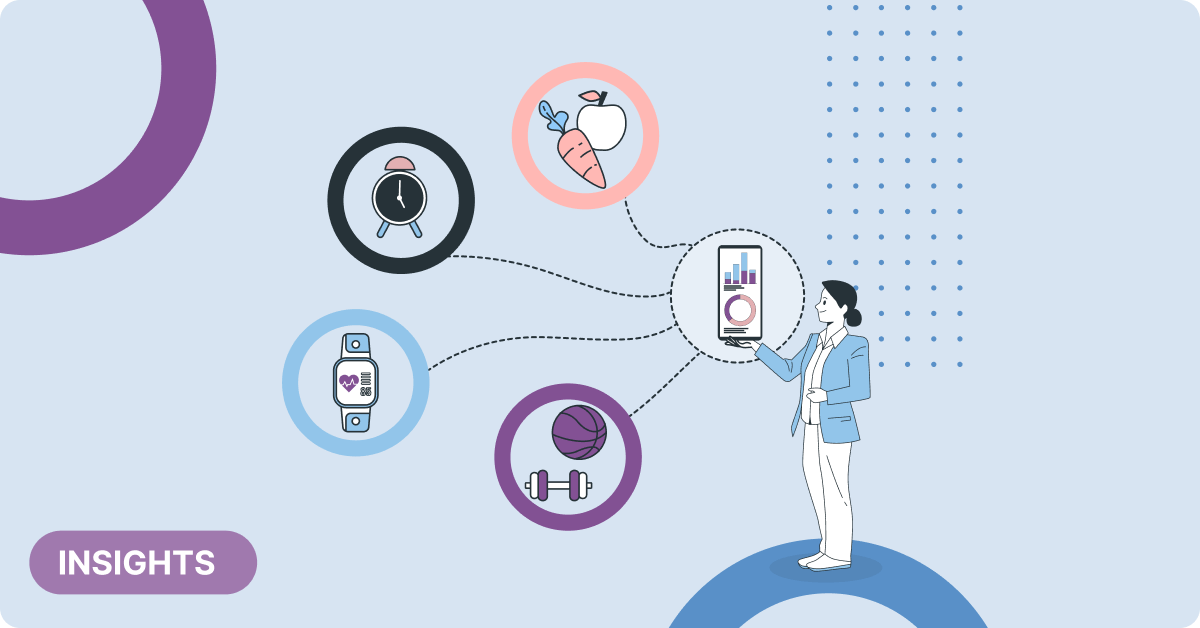
Definition & importance
Real-World Data (RWD) is any data relating to a patient’s health status, collected during the routine delivery of care, as opposed to data collected within the controlled setup of clinical trials. Hence RWD does not differ so much in its type but in the process and population involved in its collection. The different types and sources of RWD can be:
- Clinical data from electronic health records (EHRs) and case report forms (EHRs). This data establishes who the subject is, providing demographics, family history, comorbidities, procedure and treatment history, and outcomes. Such data types are also common in clinical trials.
- Patient-generated data from patient-reported outcome (PRO) questionnaires, or measurements from wearables. This is data collected in everyday setting, providing insights directly from the patient, beyond clinic visits, procedures, and hospitalization. While patient-generated data is not unusual in clinical trials, it is collected in a centralized manner at the regular visits of the trial volunteers to the healthcare facilities. In the real-world context, the collection is done continuously at home.
- Public and government data including cost and utilization data. Such data provides information on the healthcare system and the different stakeholders therein.
Such information can be used to create algorithms for risk stratification or to gain insight into associations between exposures, interventions, and outcomes.
While clinical trials continue to be the main tool for studying the safety and efficacy of a new medicine, their controlled environment, and well-defined cohorts constitute experimental conditions that do not represent real-world settings. RWD is a much better tool for understanding how patients react to a medicine once approved and made available in the market, i.e., in routine medical care. The lack of highly controlled settings usually results in lower levels of confidence, but the outcomes represent a wider population of subjects. Such outcomes are better suited for understanding and taking decisions in everyday medical care, in broader settings than the controlled ones in clinical trials.
RWD: Collecting in a clinical vs. everyday setting
There can be a huge quality difference between RWD collected in a clinical versus in everyday setting. In a clinical setting, the process is carried out sporadically by professionals, with subjects following strict guidelines (like time and method of collection, or diet prior to collection). In the everyday setting, the process is continuous and carried out by the subjects themselves. Whether the data is reported by the subjects or is measured by devices the subjects operate, the continuous nature and the self-supervision can lead to low quality due to device failure (usually uncharged devices, wearables not worn when they should have been, or mobile applications left unused for too long and automatically closed down) and lack of adherence (forgetting to answer instances of repeating questionnaires, amplified decline of interest in the process). Also, clinical data can be much more specialized to the medical conditions at hand, compared to most behavioral data collected in an everyday setting.
But no matter these shortcomings when dealing with data collected in an everyday setting, it is now well-established that behavior is part of the intervention. The high specialization and quality of the sporadic clinical data is complemented by the continuous nature of the behavioral, everyday data, in much the same way a low-resolution film complements the understanding offered by the occasional high-resolution photo.
Patient-generated, everyday RWD types
The behavioral, everyday RWD are categorized in terms of collection method and content. The following collection options are used:
- Patient-reported via questionnaires: This collection model is closer to the established clinical trial approach, but this time the questionnaires are digital, pushed to subjects via some companion mobile app. They mostly have to do with self-assessment of different aspects.
- Patient-reported via widgets: Similar to questionnaires, only this time rich graphical interfaces are employed. The widgets allow manual entry, or take advantage of integration with 3rd party devices meant for occasional use like scales or blood pressure monitors to automatically collect measurements.
- Automatically reported by wearables: Continuous measurements from wearable devices is one of the most prominent sources of RWD. Ubiquitous activity trackers or more specialized devices like sleep monitors are integrated either at device level (when a Software Development Kit is available, e.g., via Apple Health Kit) or at device cloud level (when an Application Programming Interface is available).
Using any of the above methods, the following everyday RWD types are collected:
- Physiological: Data about physical activity, continuous monitoring of vitals, sleep
- Psychological: Emotions
- Social: Interactions (phone calls, social media)
- Environmental: Living and working environment
Learning on RWD
At a raw level, RWD can lead to decisions about individuals and cohorts via analytics visualizations. But a full understanding of the context of subjects is gained via processing, using machine learning techniques. Supervised algorithms facilitate learning biomarkers, while unsupervised ones lead to phenotypes.
RWD facilitates learning digital composite biomarkers. Biomarkers are quantities characterizing some disease or outcome. Digital refers to their attributes being ubiquitously available, not only as clinical data. Composite refers to the combination of multiple attributes in an attempt to predict some outcome. ML algorithms are used to learn outcome predictors as non-linear combinations of the attributes into the digital composite biomarkers.
Phenotypes characterize the way the internal conditions of subjects manifest themselves for external observation. The different RWD attributes measured constitute the observation, and clusters of the observations correspond to different phenotypes. The clusters are learned from RWD using unsupervised ML algorithms. The clusters are then modeled for efficient representation of the phenotypes.
RWD in Healthentia
Our product Healthentia is used to collect all types of patient-generated, everyday RWD types. Our subjects employ the Healthentia mobile app to answer questionnaires and to enter data via the widgets, either manually or using devices integrated via their Software Development Kits. Data collection also employs the Healthentia big data platform and ingests more subjects’ data using the Application Programming Interfaces of other device providers.
The collected RWD is analyzed using the BI analytics available at the Healthentia portal for healthcare professionals. It is also processed using the smart services of Healthentia, namely:
- The Learning Services for training models
- The Inference Services for inferring with the help of the trained models
- The Clinical Pathway for utilizing the raw RWD and the inference results in monitoring the state of subjects, and
- The Virtual Coach for utilizing all the above in personalized advice given to the subjects.