Digital Phenotyping
Clustering of subjects into behavioral groups
Patients can be grouped together with unsupervised ML algorithms operating on their behavioral data. Hence patients within a group exhibit behavior with some distinct elements. Describing the groups with models allows on the one hand the matching of new patients in any of the groups, our digital phenotyping.
On the other hand, it enables the generation of synthetic data of similar properties, the basis of our in silico trials. If the groups also exhibit some distinct clinical outcome, the group models are validated into phenotypes.
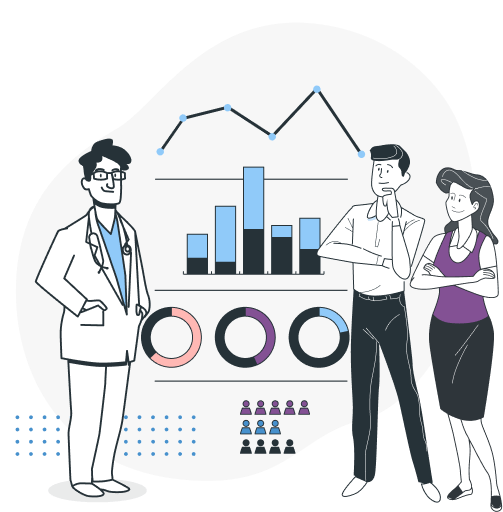
Capabilities

State-of-the-art unsupervised ML algorithms
We analyze the performance of different clustering algorithms to determine the one befitting the dataset at hand

Adaptive group modeling
Utilize Gaussian Mixture Models with adaptively determined number of Gaussians

Clinical-oriented group validation into a phenotype
Validate into phenotypes those groups whose patient members exhibit similar clinical outcomes
Clustering
Unsupervised ML algorithms exploit relations between the data of patients, clustering the data into groups. Group optimization automatically determines their number.
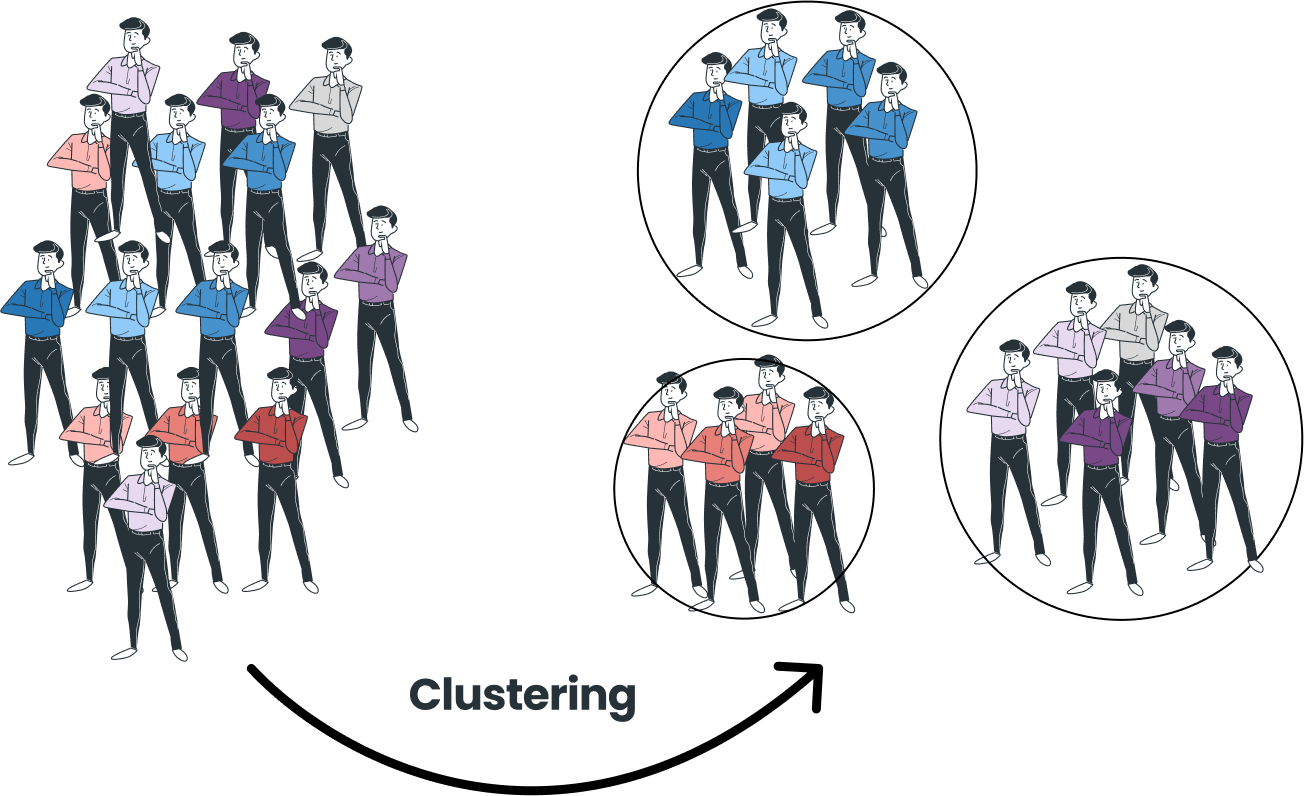
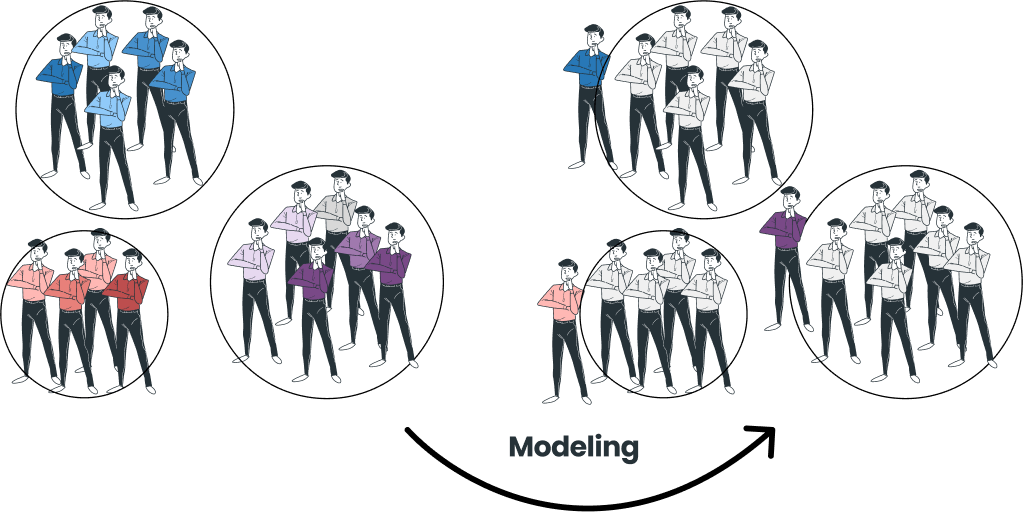
Modelling
Each group is modeled using Gaussian mixtures. The number of Gaussians describing the groups is automatically determined by an algorithm that exploits the quality of matching between the Gaussian and the data.
Phenotyping
Patients are assigned to different groups, based on matching their data in different times to the group models. To carry out the matching across time, each patient carries weights for all groups. At every matching instant the weights are updated, in the long run leading to a winning group.
Patients being in the long run assigned to a group should exhibit similar clinical outcomes. This is verified using biomarkers that predict outcomes, and this leads to a validation of groups into phenotypes.
This process of verifying groups into phenotypes and assigning people to these phenotypes is our digital phenotyping.
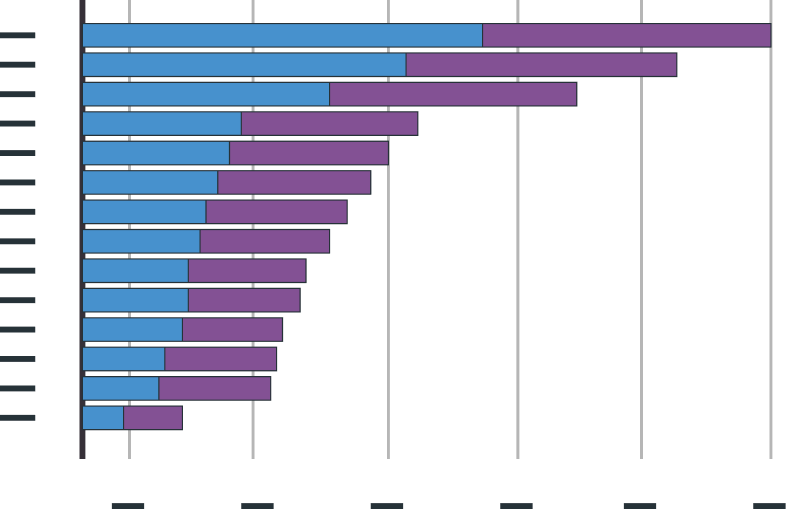