Digital Biomarkers
Measurable biomarkers indicate clinical outcomes.
Complex situations require multiple attributes to be enumerated via measurements or reports to establish the development of a clinical outcome. These attributes can be enumerated outside clinical context, using ubiquitous devices or digital questionnaires. Such biomarkers are termed digital composite ones.
Our flow for biomarker discovery utilizes Healthentia for data collection and Healthentia Smart Services to establish the important attributes that indicate any clinical outcome, as well as the way these attributes should be combined into the biomarker via learning predictive models. It facilitates data-driven and clinical validation of these models into digital composite biomarkers.
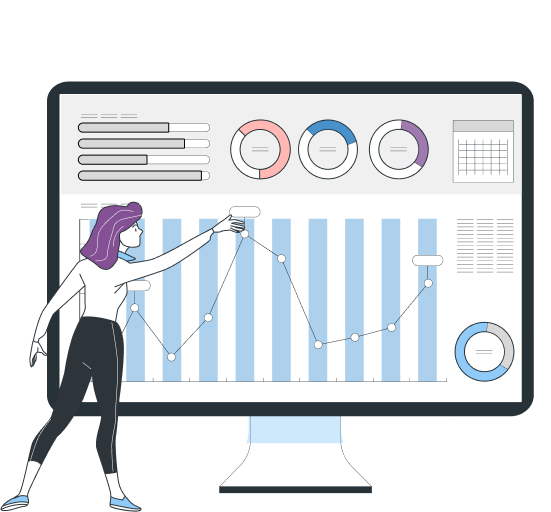
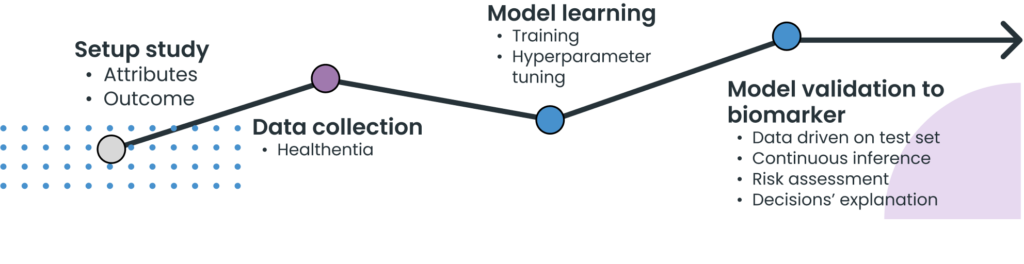
Capabilities

State-of-the-art ML algorithms
We select the best algorithm that fits the data volume and outcome type

Clinical-oriented model validation into a biomarker
Utilize held-out data to determine generalization abilities of trained model

Model hyperparameter tuning
Model learning via tuning model parameters in model training loops

Data-oriented model validation
Allow healthcare professionals to work with the model and assess its inference accuracy, risk assessment capabilities, and decision rationale in terms of attributes
Predictive modelling
Designing studies for biomarker discovery
We collaborate with healthcare professionals to establish outcomes of clinical interest and attributes that can possibly influence them.
Healthentia is used to run these studies and we process anonymized versions of the collected data to learn predictive models.
We employ the most suitable ML algorithm for your data and problem, and we perform an initial validation of the model using held-out data.
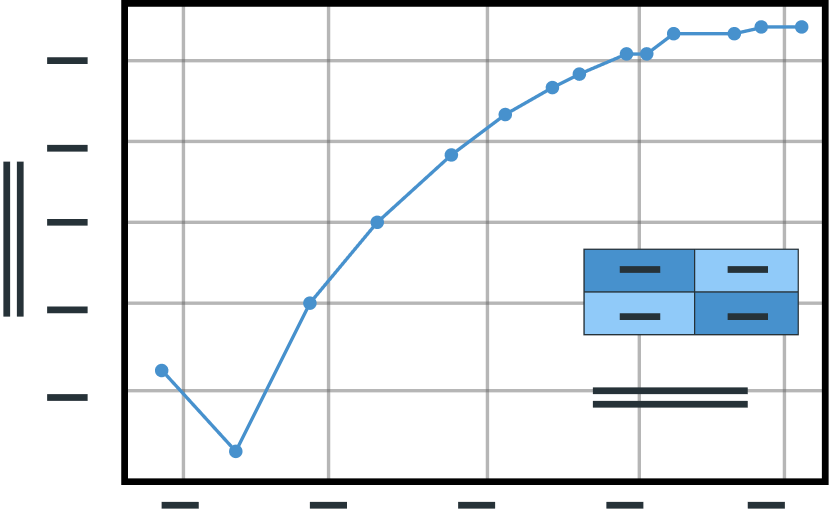
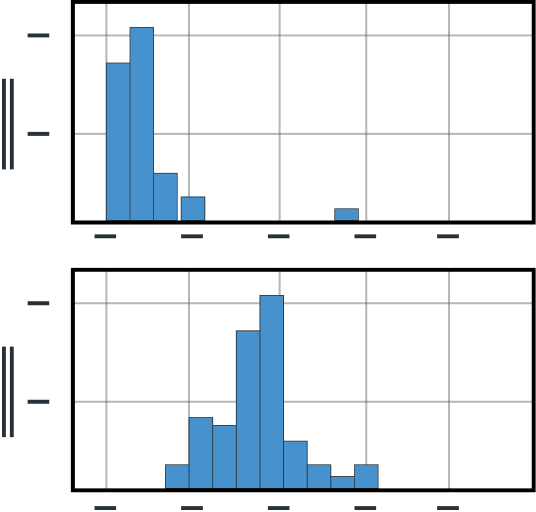
Inference & risk stratification
Infer decisions in real-time
New data is fed into the predictive models of acceptable accuracy to infer decisions in near real-time. These decisions are monitored by healthcare professionals, providing an initial clinical validation.
The decisions for one study participant are accumulated across time to characterize the participants in terms of risk. Clinical validation of the risk is a second step in validating the model into a biomarker.
Explainability
Analysis of important attributes that let to Individual decisions
Healthcare professionals can determine if the provided attribute ranking is meaningful, thus providing the final validation of the model into a biomarker for the given outcome.
The important attribute analysis in the long run indicates attributes that can be omitted from the study, making the process more GDPR compliant in terms of validating the need to measure. In a personal level and for a short term, this analysis yields important attributes of the lifestyle that need to be strengthened or changed to reduce risk, offering the basis for our personalized virtual coaching.
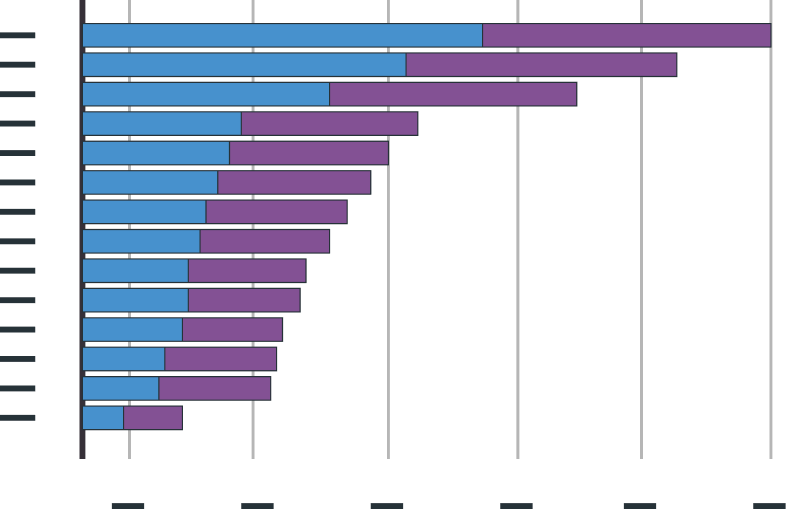